Walgreens to close 1,200 US stores in an attempt to steady operations at
home
Send a link to a friend
[October 15, 2024] By
TOM MURPHY
Walgreens plans to close about 1,200 locations over the next three years
as the drugstore chain seeks to turnaround its struggling U.S. business.
The company said Tuesday that about 500 store closures will come in the
current fiscal year and should immediately support adjusted earnings and
free cash flow. Walgreens didn't say where the store closings would take
place.
Walgreens operates about 8,500 stores in the United States and a few
thousand overseas. All of the stores that will be closed are in the
United States.
Walgreens Boots Alliance Inc. leaders said in late June that they were
finalizing a turnaround plan for its U.S. business, and that push could
result in the closing of hundreds of underperforming stores.
The plan announced Tuesday includes the closing of 300 stores that had
been approved under a previous cost-cutting plan.
Walgreens CEO Tim Wentworth said in a statement that fiscal 2025, which
began last month, will be an important “rebasing year” for the drugstore
chain.
"This turnaround will take time, but we are confident it will yield
significant financial and consumer benefits over the long term,” he
said.
Walgreens, like its competitors, has been struggling for years with
tight reimbursement for the prescriptions it sells as well as other
challenges like rising costs to operate its stores.
The Deerfield, Illinois, company also has been backing away from a plan
to add primary care clinics next to some if its stores after launching
an aggressive expansion under previous CEO Rosalind Brewer.
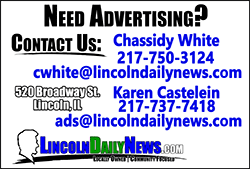
[to top of second column] |
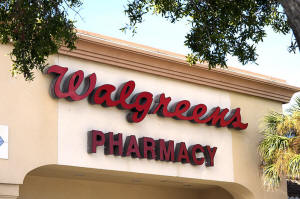
A Walgreens store in Bradenton, Fla., is shown on Feb. 9, 2024. (AP
Photo/Gene J. Puskar, File)
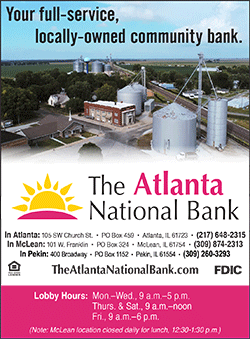 Walgreens said in August that it was
reviewing its U.S. healthcare business, and it might sell all or
part of its VillageMD clinic business. That announcement came less
than two years after the company said it would spend billions to
expand the business.
The company started 2024 by cutting the dividend it pays
shareholders to get more cash to grow its business. The drugstore
chain then slashed its forecast for fiscal 2024 in June.
Walgreens said Tuesday that its net loss swelled to more than $3
billion in the final quarter of 2024. Adjusted earnings totaled 39
cents per share, and sales grew 6% in the quarter to $37.5 billion.
That topped Wall Street expectations. Analysts expect, on average,
earnings of 36 cents per share on $35.75 billion in revenue in the
fiscal fourth quarter, according to FactSet.
The company also said it expects adjusted earnings in the new fiscal
year to fall between $1.40 and $1.80 per share, with growth in its
U.S. healthcare and international businesses countering the U.S.
retail pharmacy decline.
For the fiscal 2025, analysts expect adjusted earnings of $1.72 per
share.
Walgreens shares jumped 5% Tuesday in early morning trading.
The stock had shed nearly two thirds of their value so far this
year, falling to $9 as of Monday’s close.
All contents © copyright 2024 Associated Press. All rights reserved |